
CONFERENCE TO BE HELD IN
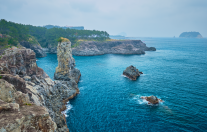
Jeju Island, South Korea

CO-SPONSORED BY
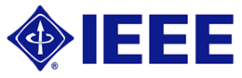
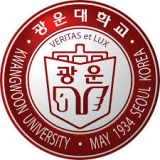
PATRONS
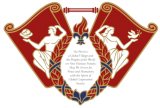
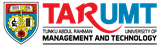
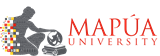
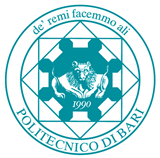
|
KEYNOTE
SPEAKERS

Prof. Jin Ho Chang
Daegu Gyeongbuk Institute of Science and Technology (DGIST),
Republic of Korea
Jin Ho Chang is the Dean of the College
of Transdisciplinary Studies at Daegu Gyeongbuk Institute of
Science and Technology (DGIST) and a Professor in the
Department of Electrical Engineering and Computer Science.
He earned his M.S. and B.S. degrees in Electrical
Engineering from Sogang University in 2002 and 2000,
respectively, and received his Ph.D. in Biomedical
Engineering from the University of Southern California in
2007. Prior to joining DGIST, he served as a faculty member
at Sogang University from 2010 to 2020, progressing from
Assistant Professor to Full Professor. He has held key
leadership positions, including serving as an executive
member of the Korea Engineering Deans Council, a board
member of the National Daegu Science Museum, a member of the
Medical Device Review Committee of the Ministry of Food and
Drug Safety, and a review board member of the National
Research Foundation of Korea. He is also actively involved
in various professional organizations, including the Korean
Society of Medical & Biological Engineering and the Korean
Society for Therapeutic Ultrasound. Additionally, he served
as an Associate Editor for IEEE Transactions on UFFC from
2014 to 2022. His research focuses on medical ultrasound
imaging and therapy, ultrasound-optical fusion technology,
and deep learning for medical imaging. He has published over
85 SCI journal articles, including in top-tier journals such
as Nature Photonics and Nano Energy, and holds more than 75
registered patents, with technology transfers exceeding 1.2
billion KRW.
Speech Title: "Ultrasound-Induced Optical Clearing for Deep Optical Imaging and Photothermal Therapy"
Abstract: Hybrid imaging techniques have
garnered significant attention, as each medical imaging
modality has inherent strengths and limitations. Combining
modalities allows the simultaneous acquisition of
complementary information, enhancing diagnostic
capabilities. Among various imaging modalities, ultrasound
and optical imaging are notably compatible, each
complementing the other's advantages and mitigating
respective limitations. Consequently, numerous studies have
explored ultrasound-optical hybrid systems to generate novel
clinical value. Additionally, ultrasound has facilitated
advanced optical imaging methods designed to overcome the
inherent limitations of light penetration in biological
tissues, notably through techniques like
ultrasound-generated guidestars for wavefront shaping. In a
distinctly different approach, our research team recently
introduced ultrasound-induced optical clearing (US-OC), a
groundbreaking method that leverages transient gas bubbles
generated by ultrasound along the optical path to
significantly reduce tissue optical scattering. We
successfully applied US-OC to laser scanning microscopy
(US-OCM), demonstrating over a six-fold increase in imaging
depth compared to conventional methods, while preserving
imaging resolution (Nature Photonics, vol. 16, 762-769,
2022). We also have applied US-OC to photothermal therapy
(PTT), recently published in Advanced Optical Materials
(2400332, 2024). PTT selectively delivers laser energy to
chromophores within targeted lesions, minimizing side
effects. However, its clinical applicability has been
restricted by limited therapeutic depth due to optical
scattering. To overcome this barrier, we developed
ultrasound-induced optical clearing-assisted PTT
(ULTRA-PTT). In this presentation, I will discuss the
foundational principles and potential clinical applications
of US-OC, US-OCM, and ULTRA-PTT.
Prof.
Nagendra Kaushik
Kwangwoon
University, South Korea
Prof. Nagendra Kaushik is working at the
Department of Electrical and Biological
Physics & Plasma Bioscience Research Center
at Kwangwoon University, Seoul (South Korea)
since 2011. His research work is primarily
focused on plasma bioscience & medicine,
plasma agriculture, plasma environment,
cancer biology & immuno-modulations, plasma
chemistry, nanobiotechnology, and
biomaterials. He has published more than 150
high impact publications, including many in
top-ranked journals such as Biomaterials
(impact factor 15.6), Cancer Research
(impact factor13.3), Journal of Advanced
Research (impact factor 12.4), Green
Chemistry (impact factor 11.3), Materials
Today Bio (impact factor 10.7), Science of
The Total Environment (impact factor 10.75)
and Journal of Nanobiotechnology (impact
factor 11.5) and Bioactive Materials (impact
factor 17.3) and applied several product and
process patents. His H-index is 34 and the
I10 index is 74 with a total citation of
around 5000. Prof. Nagendra is serving as
the editor of more than 20 journals
including Scientific Report, PloS One, IEEE
Journals, Frontiers journals, and many
others. He is also listed in World's Top 2%
Scientists by Stanford University and
Elsevier continuously since 2020.
INVITED
SPEAKERS

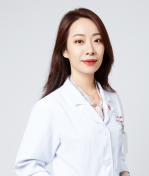
Dr. Huiping Luo
Fudan University, China
Dr. Luo is the Associate Chief Physician of
the Department of Otolaryngology, and also a Licensed Mental
Health Counselor-Intermediate Level. She is the PGME alumni as
a Harvard University GCSRT and T2T member, and a Visiting
Scholar at the University of Wisconsin Madison. Dr. Luo is
member of the China Science Writers Association, and director
of the Sleep and Respiratory Disorders Diagnosis and Treatment
Alliance. Her main research interests include sleep-disordered
breathing, the management of chronic ENT diseases, and related
psychological therapy. Dr. Luo is also co-PIs in many
interdisciplinary researches, which extensively involve
daytime speech analysis, snore sound analysis, the development
of prediction system for OSA upper airway surgery and online
diagnosis system for OSA based on artificial intelligence.
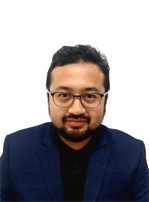
Dr. Mohd Khairul Izamil
University of Malaysia Pahang Al-Sultan
Abdullah, Malaysia
Dr. Mohd Khairul Izamil is a neuroscientist
and medical doctor with expertise in neuroscience,
neuroimaging, occupational health, and epidemiology. He
received his Ph.D. in Neuroscience with First Class Honours
from Universiti Sains Malaysia in 2020 and an MBBCh from the
University of Cairo in 2013. His research focuses on brain
structural changes related to workplace stress and
neurological disorders, using advanced MRI techniques,
particularly diffusion tensor imaging (DTI), to study white
matter integrity and neural connectivity. He has contributed
significantly to understanding the neurobiological effects of
occupational stress, depression, noise-induced hearing loss
(NIHL), and speech-in-noise processing. Dr. Izamil has
received research funding from UMPSA, the National Institute
of Occupational Safety and Health (NIOSH), and Malaysia's
Ministry of Higher Education. He has also earned several Best
Presenter Awards at national conferences. Currently, he is a
Senior Lecturer at UMPSA, teaching occupational epidemiology,
human anatomy, and physiology. He frequently speaks at
international conferences and reviews for scientific journals.
He also promotes science communication through national media
articles on neurorehabilitation, occupational health, and
cognitive neuroscience. Dr. Izamil is dedicated to advancing
mental health and cognitive well-being in work environments
through evidence-based neuroscience and neuroimaging research.
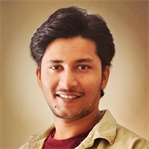
Dr. Rajan Prasad
Khalifa University, UAE
Dr. Rajan Prasad is a Postdoctoral Fellow
in the Department of Mechanical and Nuclear Engineering at
Khalifa University, Abu Dhabi, UAE. His research integrates
mechanical design, biomedical systems, and robotics, focusing
on assistive technologies for rehabilitation. He completed his
Ph.D. in Mechanical Engineering with a specialization in
bio-robotics, developing a simulation-based framework for a
cable-driven lower limb rehabilitation exoskeleton (C-LREX) to
support post-stroke gait recovery—available on GitHub:
https://github.com/rajanprasad460/C-LREX-Tool. Previously, he
served as a Postdoctoral Fellow in the Medical Sciences
department at Khalifa University, conducting biofeedback
research using biosignals such as ECG, EEG, PPG, and heart
rate variability for stress reduction. He holds a master’s
degree from the Beijing Institute of Technology, China, and a
bachelor’s from IOE, Tribhuvan University, Nepal. His
interests span robotics for rehabilitation, ground,
underwater, and space systems.
|